Generative AI in data visualization:
Redefining insights in the pharma sector
🌟 Abstract
Generative AI is redefining how we visualize data in the pharmaceutical industry, providing innovative tools that unlock new avenues for insight and strategic decision-making.
🔍 Introduction
In today’s data-driven world, the ability to visualize complex information effectively is paramount. The pharmaceutical industry generates vast amounts of data from clinical trials, research studies, and market analyses. Traditional visualization methods often fall short in conveying the intricacies of this data. Enter Generative AI—an advanced technology that creates dynamic, interactive visual representations, enabling stakeholders to derive actionable insights more efficiently.
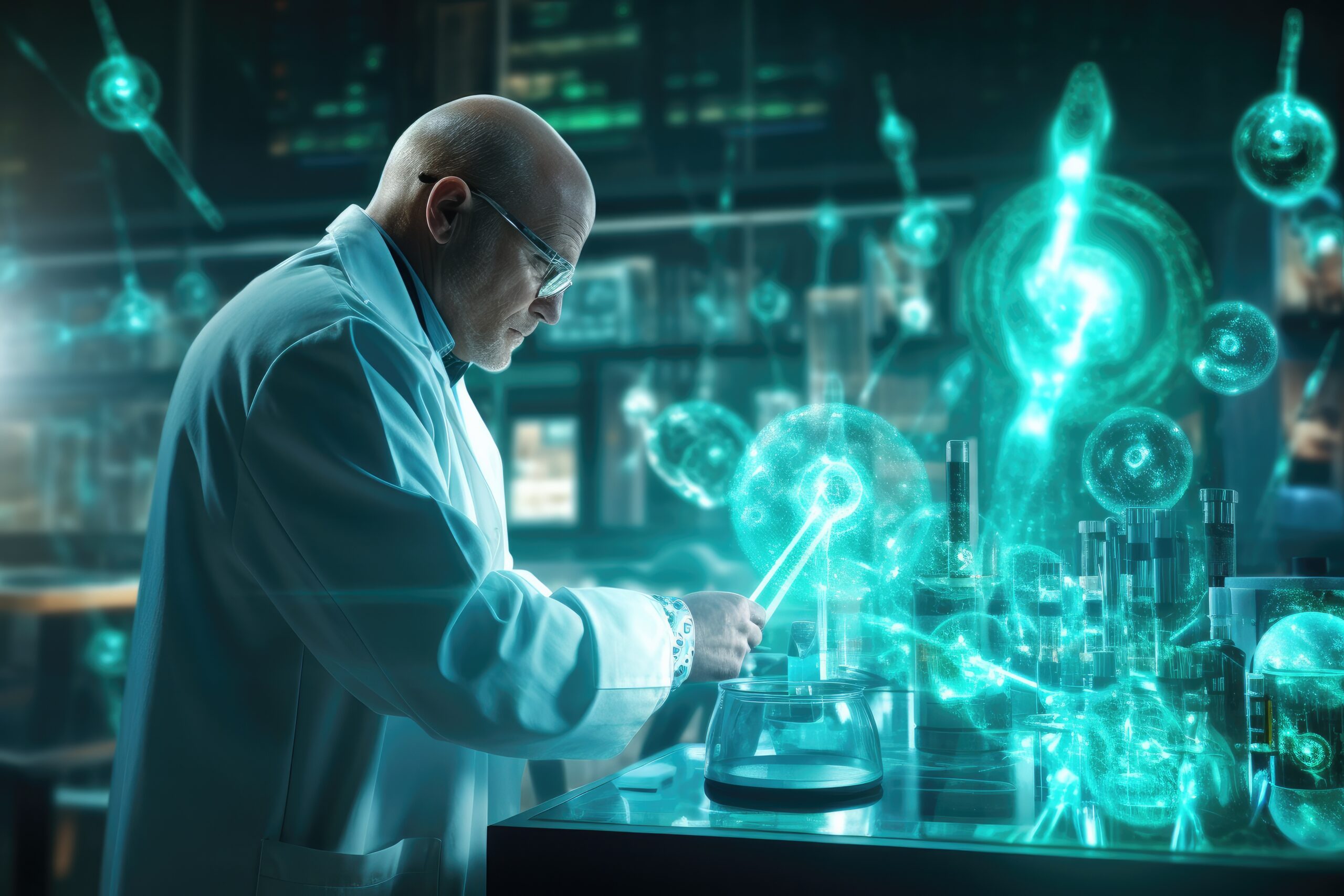
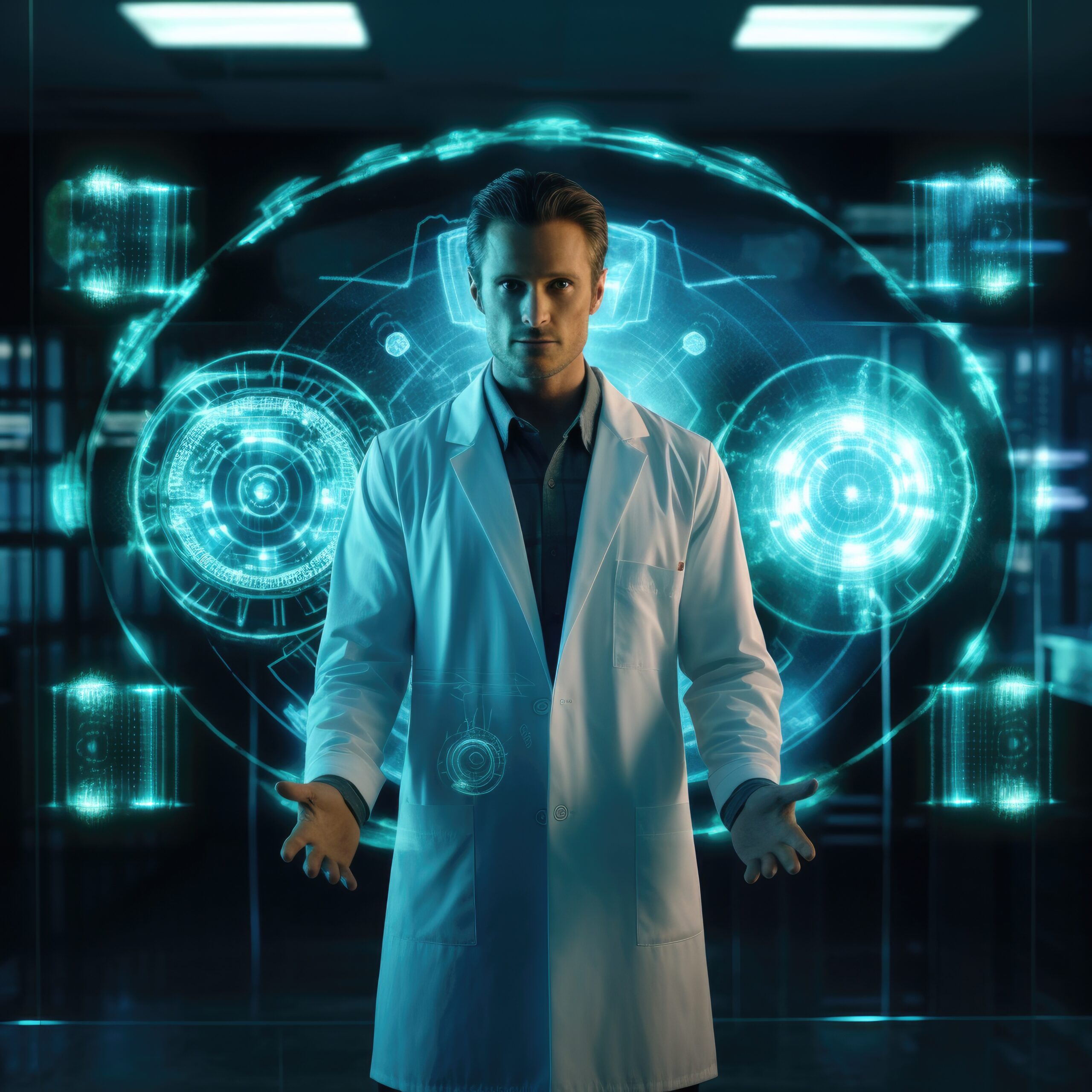
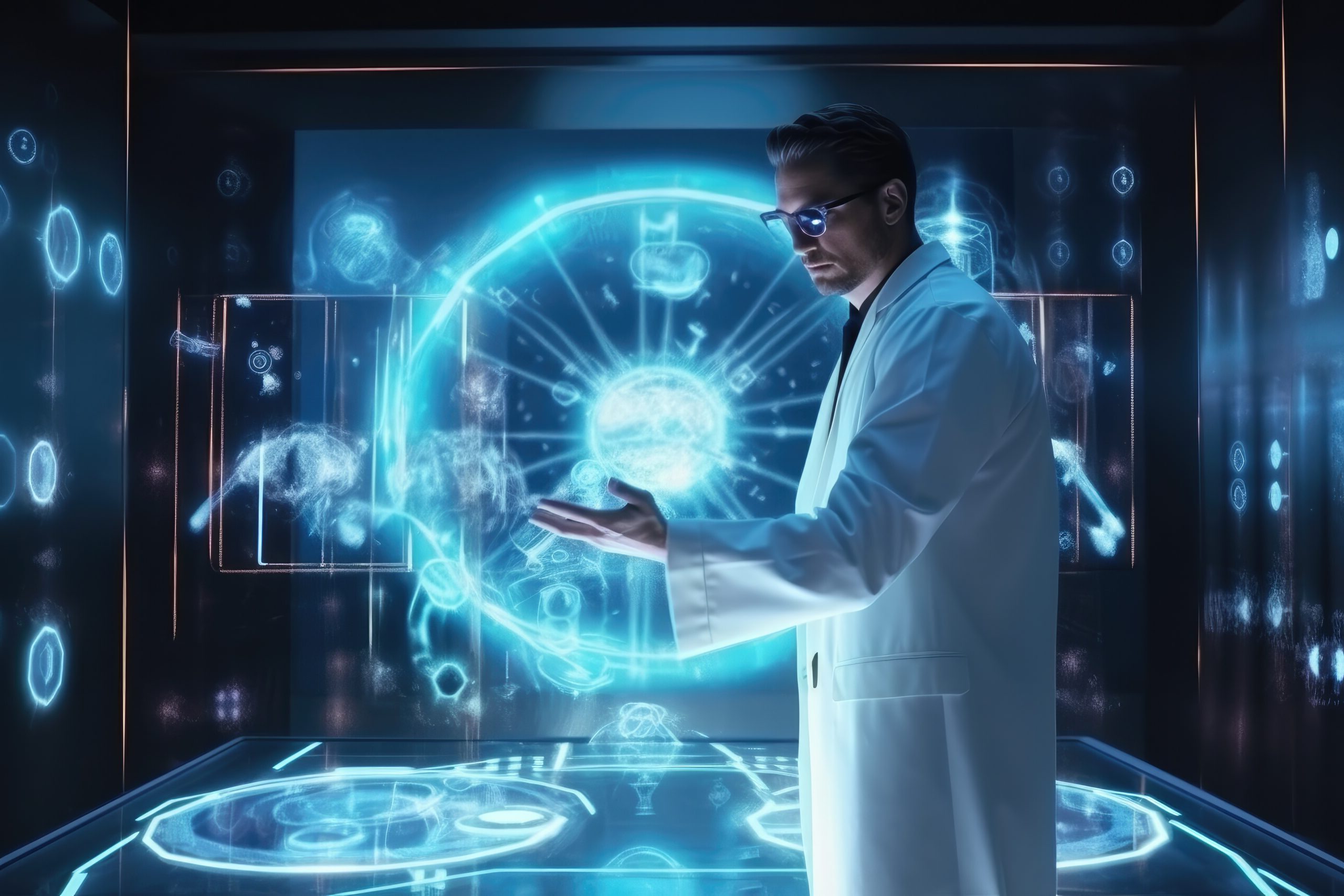
Applications of generative AI in data visualization
Enhanced Data Representation:
Generative AI allows for the creation of intricate visualizations that can adapt in real-time based on user interactions. This dynamic capability enables stakeholders to explore data from multiple angles, making it easier to identify trends, correlations, and anomalies. For example, AI-driven visualizations can illustrate patient demographics in clinical trials, highlighting variations in treatment responses across different groups.
Automated Reporting:
One of the most time-consuming aspects of data analysis is generating reports. Generative AI automates the creation of dashboards and visual reports, significantly reducing the time and effort required. By integrating real-time data feeds, these automated systems provide up-to-date insights that can be shared with stakeholders instantly. This capability empowers teams to focus on interpretation and strategy rather than data preparation.
Scenario Simulation:
Generative AI tools can model different scenarios by analyzing various parameters and their potential outcomes. For instance, in drug development, AI can simulate how changes in dosage or patient demographics might impact clinical trial results. By visually representing these scenarios, stakeholders can assess risks and opportunities, facilitating informed decision-making.
Interactive Dashboards:
Generative AI can create interactive dashboards that allow users to manipulate data visualizations directly. This interactivity enhances user engagement and provides a more intuitive understanding of complex datasets. Stakeholders can drill down into specific areas of interest, uncovering insights that may not be apparent in static visualizations.
⚠️ Challenges and future directions
While the potential of Generative AI in data visualization is significant, several challenges must be addressed:
Data Quality and Consistency:
For Generative AI to be effective, the underlying data must be of high quality and consistent. Ensuring data integrity is a critical challenge that organizations must overcome, particularly in a sector as regulated as pharmaceuticals.
User Adoption and Training:
The success of any new technology hinges on user acceptance. Pharmaceutical companies need to invest in training programs to help teams understand how to leverage Generative AI tools effectively. This includes fostering a culture of data literacy, where employees at all levels can engage with data confidently.
Interpretability of AI-Generated Visualizations:
As Generative AI creates complex visualizations, it is essential to ensure that users can interpret the results accurately. Providing context and explanatory insights alongside visualizations can help bridge this gap.