Machine learning-driven energy demand optimization reduces costs by 70% and credit back losses by 45% for leading US energy provider
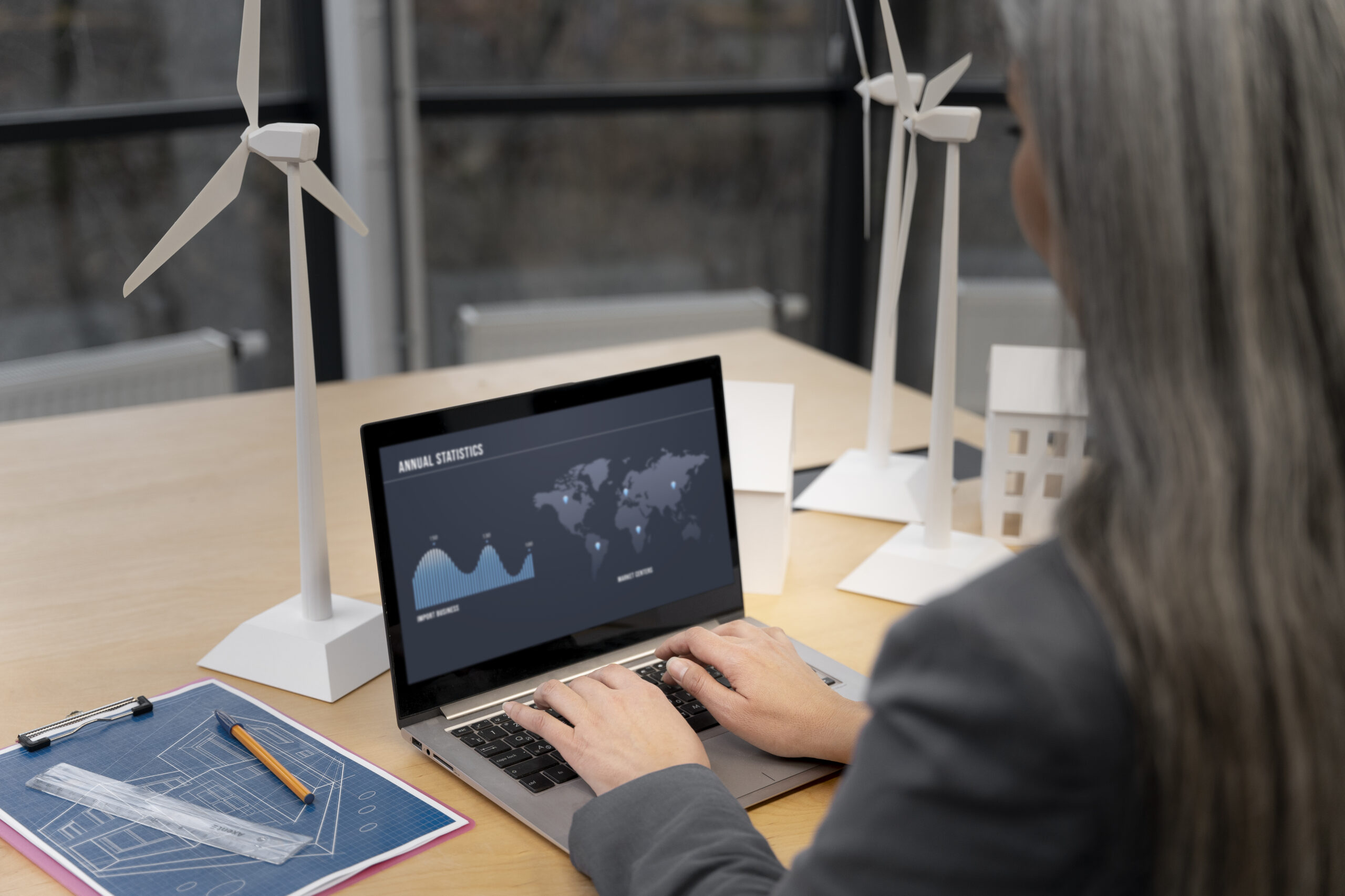
A leading US energy provider partnered with Fuld & Company to enhance its energy demand forecasting using machine learning. By combining historical energy data with weather forecasts, we optimized their predictions of energy consumption, resulting in significant cost savings and a reduction in penalty payments and credit back losses.
Key Achievements:
- 70% Reduction in Energy Costs: More accurate demand predictions led to a 70% reduction in overall energy costs by reducing energy purchases at peak prices.
- 45% Reduction in Credit Back Losses: Improved demand forecasting minimized excess energy purchases, reducing credit back losses by 45%.
- Optimized Energy Procurement: The provider was able to better align energy purchasing decisions with actual demand, avoiding penalties for demand forecasting errors.
How We Did It
Fuld & Company’s solution involved:
- Historical Energy Data Analysis: We examined past consumption trends to build a strong foundation for future demand predictions.
- Weather Forecast Integration: We incorporated real-time weather data, adjusting for temperature and other meteorological factors impacting energy demand.
- Machine Learning for Demand Prediction: Our machine learning model continuously improved predictions by learning from historical data and real-time weather inputs.
- Penalty and Loss Minimization: More accurate forecasts reduced the risk of penalties for over- or underestimating demand, saving the provider substantial costs.
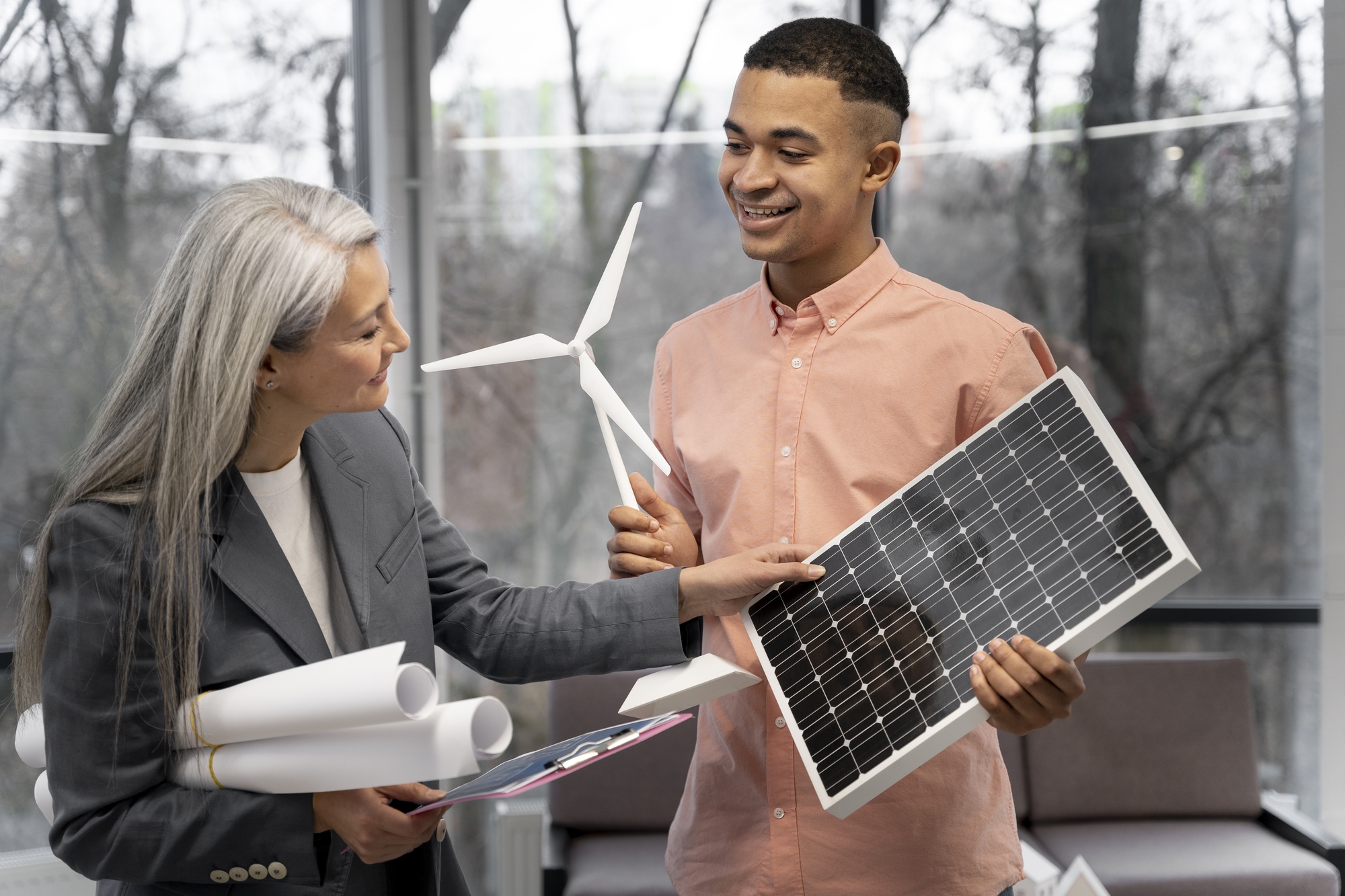