Enhancing manufacturing efficiency with predictive maintenance
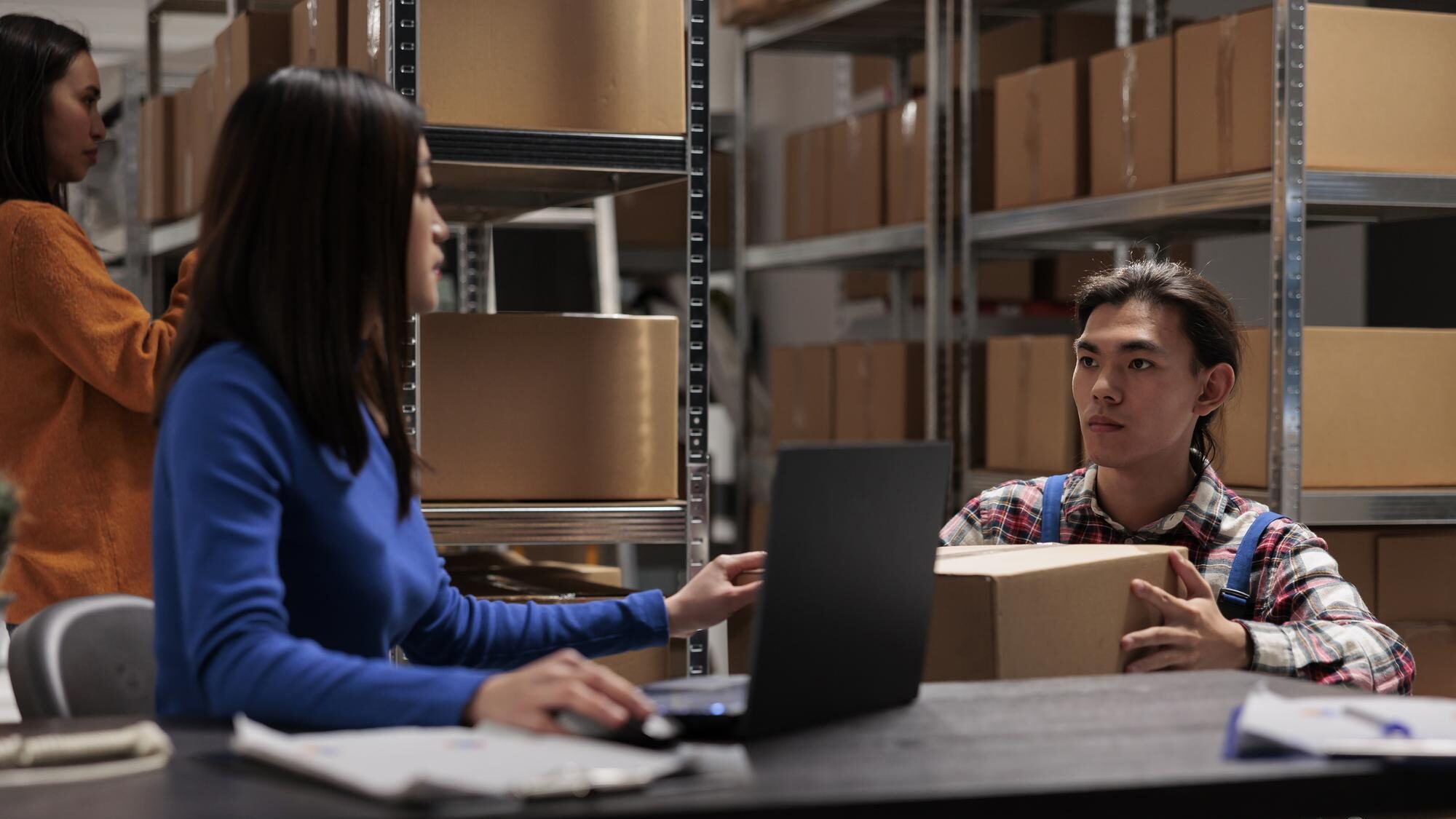
A leading US-based nutraceuticals company recognized the need to optimize its tablet manufacturing operations as expansion led to increasing production interruptions. Unplanned disruptions from machine breakdowns and maintenance issues were affecting productivity, increasing operational costs, and causing significant downtime and wastage. To address these challenges, the company implemented a predictive maintenance solution using past maintenance and operational data to anticipate machine failures. The aim was to reduce downtime, optimize maintenance schedules, and create a more efficient, cost-effective process, ultimately improving operational efficiency and profitability across its global manufacturing units.
How We Did It
Fuld & Company took a data-driven approach by implementing predictive maintenance across its tablet production lines. Here’s how the process unfolded:
- Analyzed historical maintenance data: Identified critical machines prone to failure, ensuring that focus was placed on the most impactful assets.
- Selected pilot manufacturing units: Chose three global units and identified eight high-risk machines based on past failure patterns.
- Collected sensor data: Monitored key parameters like temperature, vibration, noise, and more to understand operational conditions and detect early failure signs.
- Set up Big Data clusters: Established infrastructure for storing and processing the streaming sensor data in real-time, ensuring quick access for predictive modeling.
- Developed AI models: Used neural networks to predict failure patterns and recommend optimized maintenance schedules, improving machine reliability.
- Self-learning models: Over time, the models continuously improved, leading to a 65% reduction in downtime within one year.
- Improved production scheduling: By reducing unplanned disruptions, the company could better plan manufacturing processes, enhancing overall efficiency and profitability.
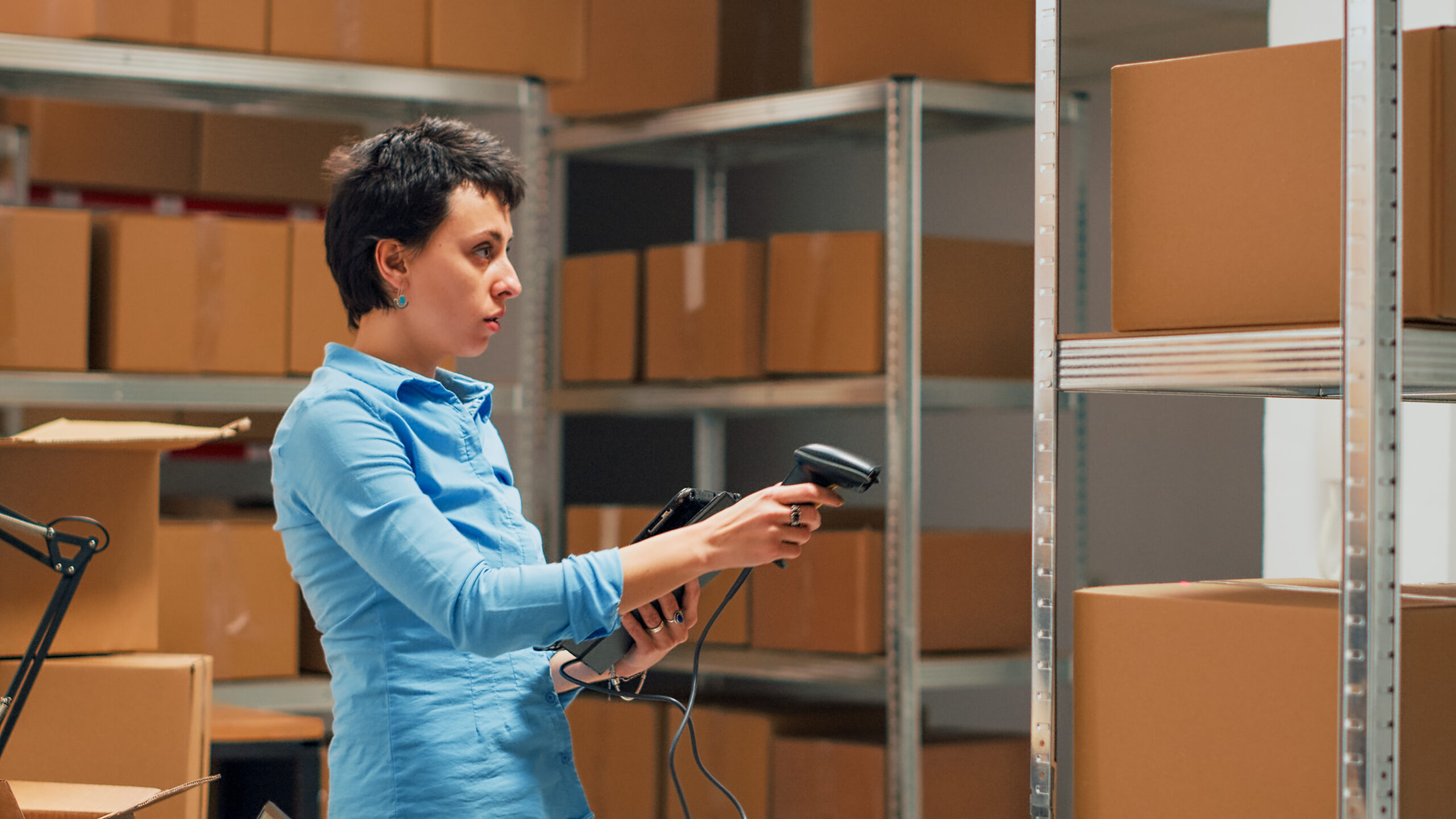