Harnessing AI in the pharmaceutical Industry:
Transforming drug discovery and development
🌟 Abstract
The pharmaceutical industry faces relentless pressure to innovate rapidly and efficiently. Artificial Intelligence (AI) is emerging as a transformative force, reshaping drug discovery and development by optimizing processes and enhancing decision-making.
🔍 Introduction
The traditional pathway for drug discovery is often long and costly, frequently taking over a decade and exceeding $2 billion per successful compound. As the complexity of diseases increases and patient needs evolve, pharmaceutical companies are turning to AI to streamline these processes. AI has the potential to analyze vast amounts of data quickly, offering insights that human researchers may overlook.
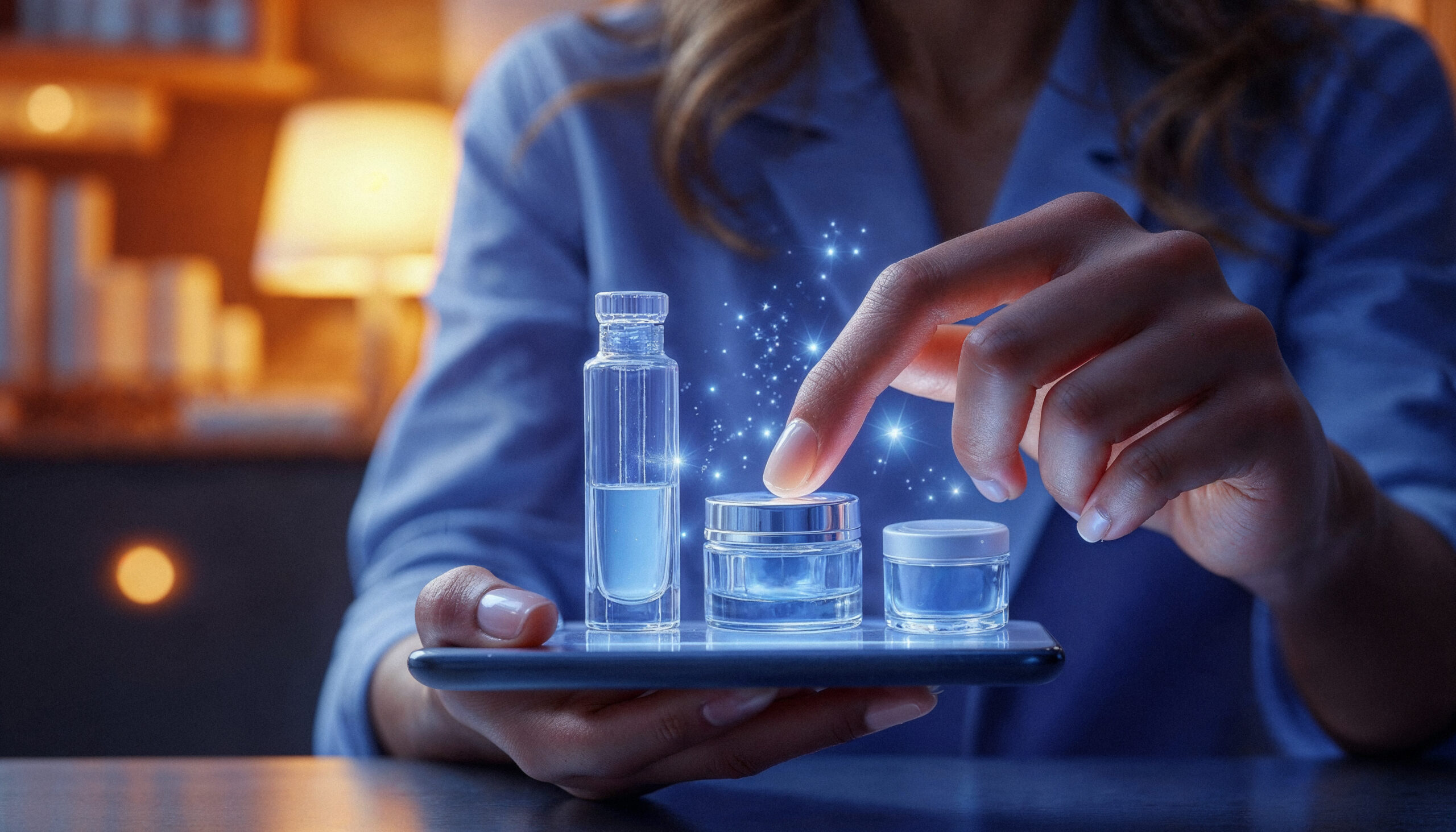
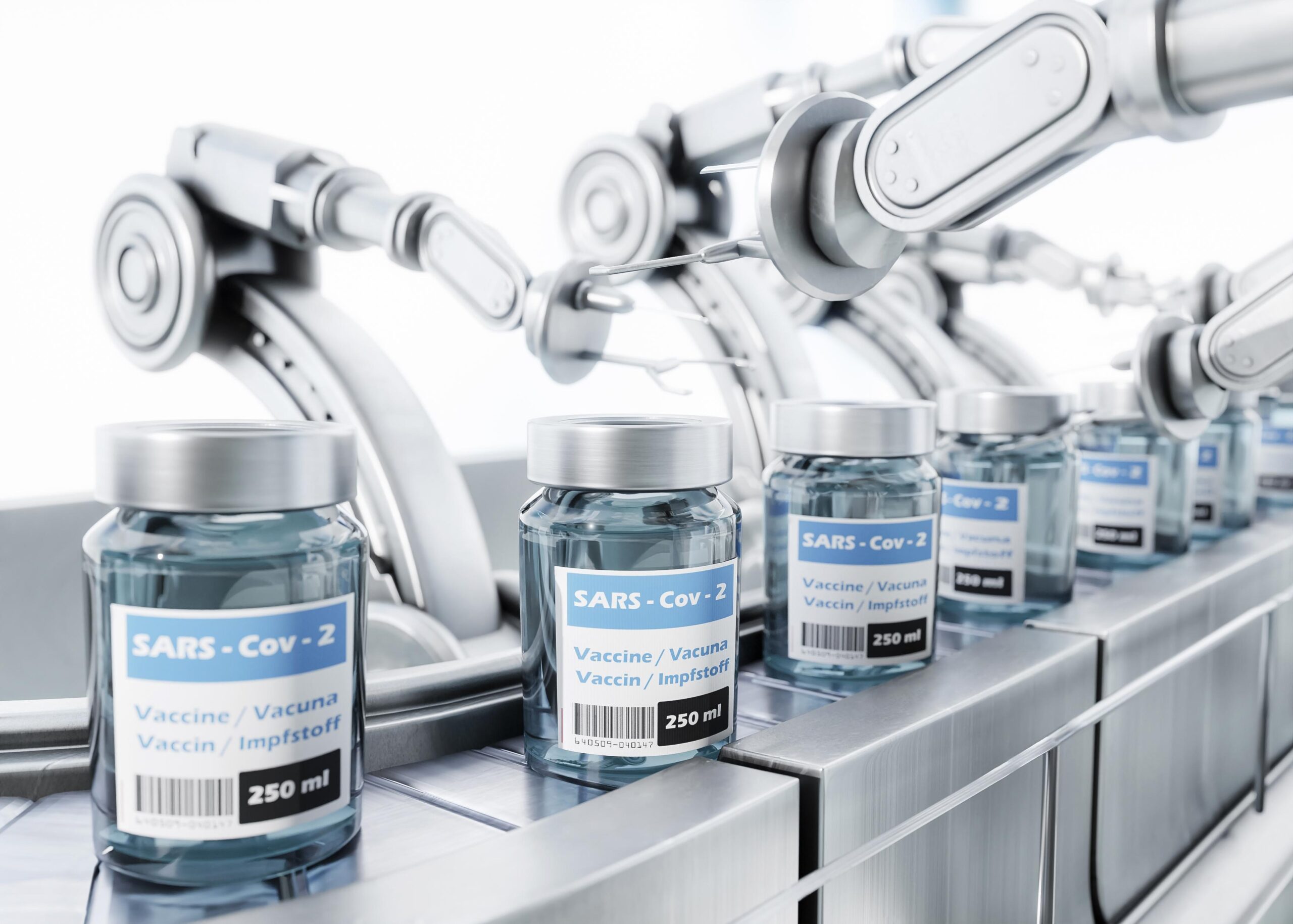
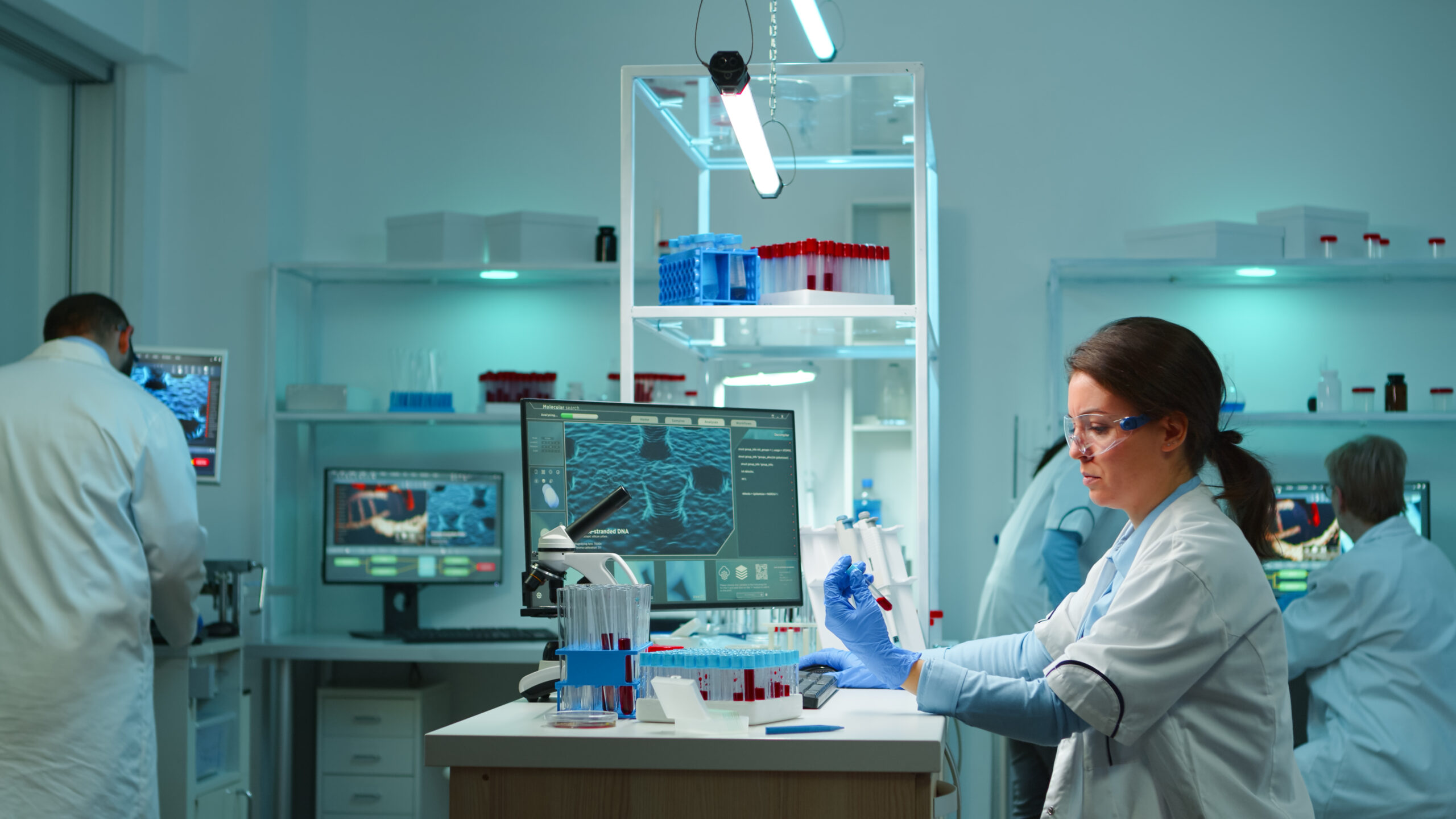
Key applications of AI in pharma
Predictive Analytics:
AI algorithms can analyze historical data from previous drug trials to forecast the success rates of new candidates. By identifying patterns and correlations, AI helps researchers prioritize the most promising compounds. This predictive capability not only accelerates the discovery process but also reduces the risk of costly failures.
Clinical Trial Optimization:
Clinical trials are essential for validating new drugs, but they are often plagued by inefficiencies. AI can optimize patient recruitment by mining electronic health records and genomics databases to identify suitable candidates based on specific criteria. This targeted approach enhances patient enrollment rates and improves the likelihood of trial success. Moreover, AI can assist in designing trials by simulating various scenarios, enabling researchers to choose the most effective trial designs upfront.
Personalized Medicine:
The future of healthcare lies in personalization. AI enables pharmaceutical companies to analyze genetic and molecular data, tailoring treatments to individual patient profiles. This approach not only improves treatment efficacy but also minimizes adverse effects, as therapies can be customized to target specific biomarkers or genetic variations. As a result, patients receive therapies that are more likely to succeed, ultimately leading to better health outcomes.
Drug Repurposing:
AI can also play a pivotal role in drug repurposing—identifying existing drugs that can be used for new therapeutic indications. By analyzing diverse datasets, AI can uncover unexpected drug-disease relationships, reducing the time and cost associated with bringing new treatments to market.
Challenges and considerations
While the benefits of AI in pharma are substantial, several challenges must be addressed for successful implementation:
Data Quality and Accessibility:
The effectiveness of AI algorithms hinges on the quality and quantity of data. Many pharmaceutical companies struggle with data silos and inconsistent data formats. Ensuring access to high-quality, structured data is crucial for AI to yield meaningful insights.
Regulatory Compliance:
The regulatory landscape for AI in healthcare is still evolving. Pharmaceutical companies must navigate complex regulatory frameworks to ensure their AI applications comply with industry standards. This requires collaboration with regulatory bodies and a deep understanding of compliance requirements.
Interdisciplinary Collaboration:
Successful AI integration necessitates collaboration among diverse teams, including data scientists, clinicians, and regulatory experts. Fostering an environment of interdisciplinary cooperation is essential for maximizing AI’s potential in drug development.