Machine learning-driven pricing optimization increases efficiency for US retail giant
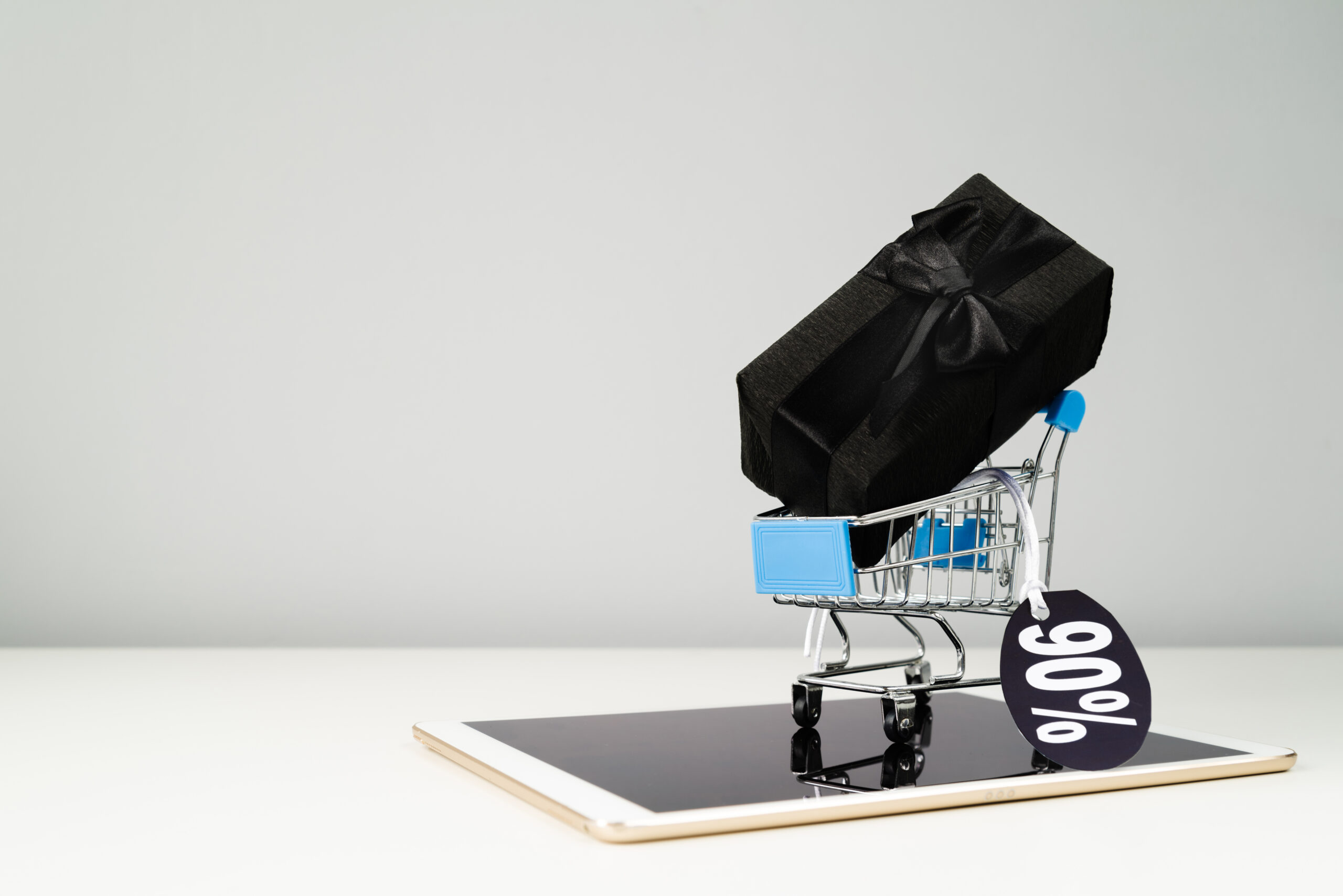
A leading US retail giant partnered with Fuld & Company to implement an advanced pricing optimization solution. By leveraging machine learning algorithms to predict the most effective pricing strategies across items and store clusters, we integrated competitive pricing data and various business rules to deliver dynamic, data-driven pricing decisions. This approach led to significant operational efficiencies and optimized pricing across a vast range of products.
Fuld’s Machine Learning-Driven Pricing Optimization Produced Impressive Results:
- 100+ Stores Optimized Daily: The pricing system optimized prices for over 100 stores each day, ensuring up-to-date and competitive pricing in real-time.
- 40K+ Items Optimized Weekly: Over 40,000 items were optimized each week, with machine learning refining predictions based on new sales data, customer behavior, and market trends.
- Improved Competitive Positioning: By integrating real-time competitor pricing data and optimizing prices across different store clusters, the retail giant maintained its competitive edge while maximizing profitability.
How We Did It
Fuld & Company designed a comprehensive pricing optimization strategy to enhance pricing decisions and drive operational efficiency:
- Machine Learning for Predictive Pricing
We implemented machine learning algorithms to predict optimal pricing for products across various store clusters. By analyzing historical sales data, customer behavior, and pricing trends, the system dynamically adjusted prices based on factors like demand fluctuations, seasonality, and promotions.
- Competitive Pricing Integration
We integrated real-time competitor pricing data into the model to ensure that the retail giant’s prices remained competitive while maximizing profit margins. The model continuously adjusted prices based on the competitive landscape, ensuring the company stayed ahead of the competition.
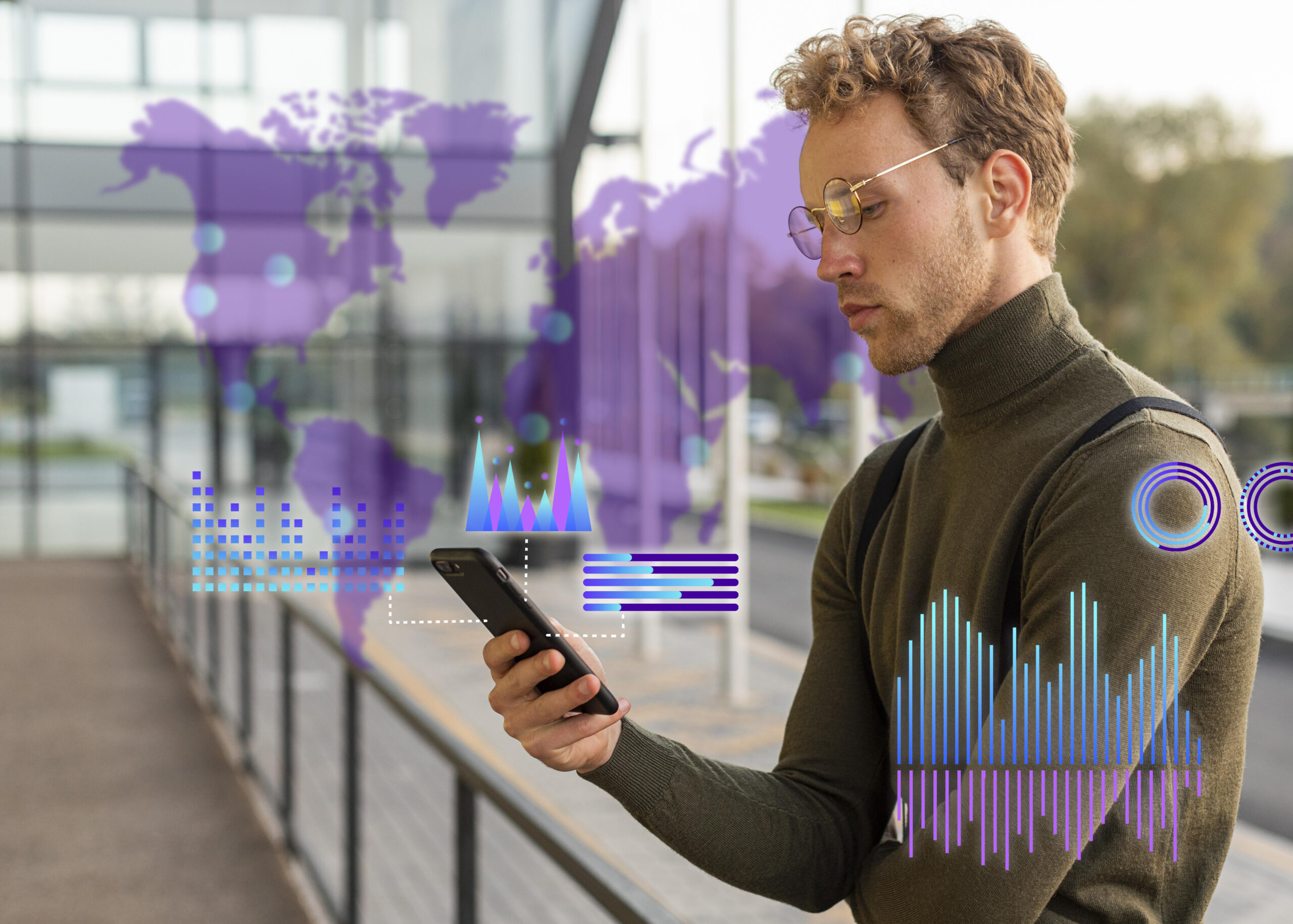
- Business Rules and Constraints
Specific business rules, such as pricing thresholds, margin requirements, and promotional guidelines, were integrated into the model. These rules ensured that automated pricing adjustments adhered to the company’s financial goals and brand positioning, while still allowing for flexibility in response to market conditions.
- Store Cluster-Level Optimization
The pricing optimization solution was designed to adjust pricing on a store-cluster level, taking regional demand and local market conditions into account. This allowed for location-based pricing strategies that ensured prices were appropriate for each geographic market, while maintaining a unified pricing structure across all stores.