Transforming policy retention with predictive analytics
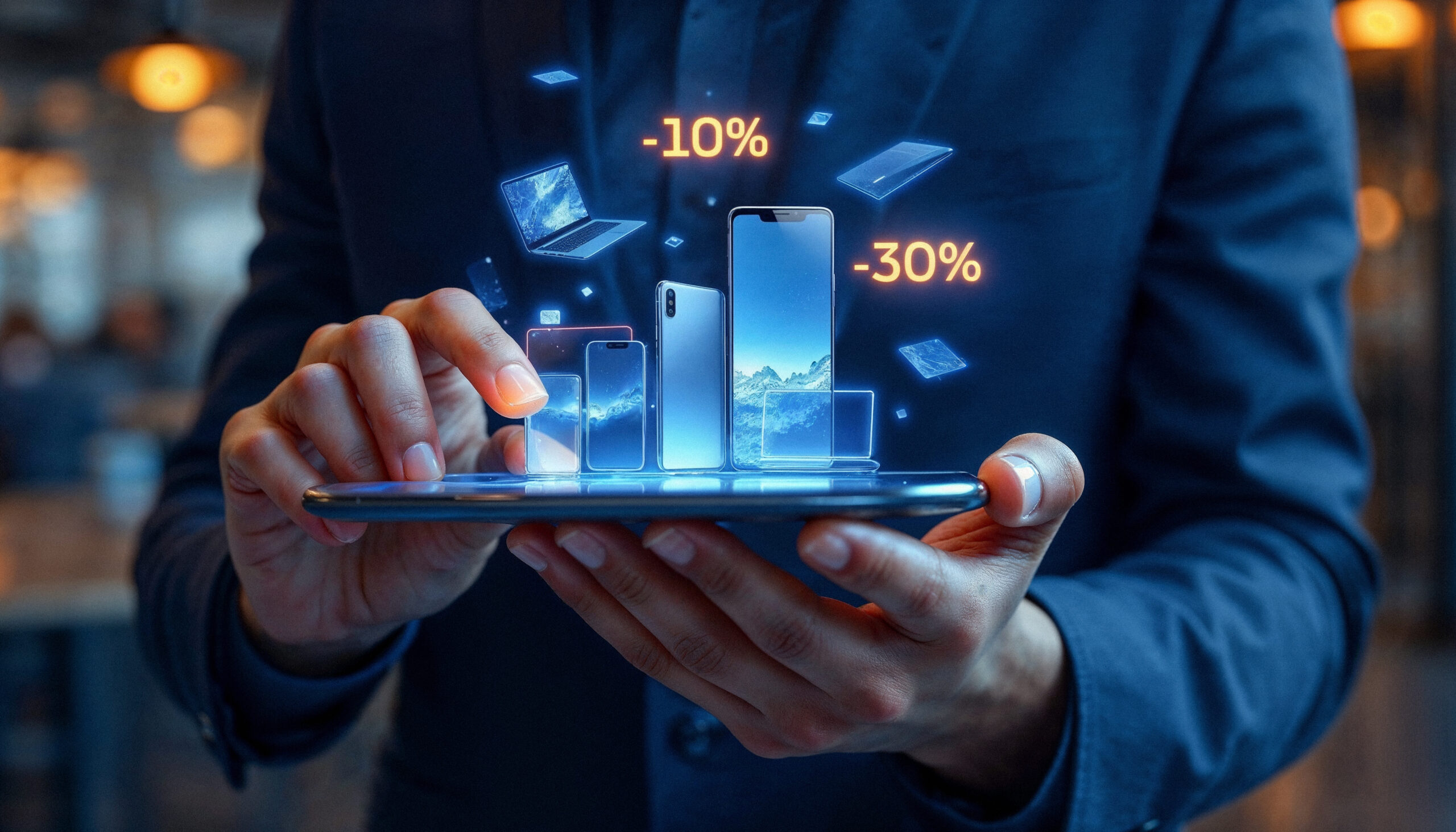
A leading South African insurer faced declining revenue and customer satisfaction due to high policy lapses, cancellations, and non-take-up rates. The company aimed to improve customer retention and profitability by leveraging predictive analytics. The objective was to identify at-risk policies, allowing for proactive interventions. By analyzing customer and policy data, predictive models helped pinpoint patterns indicating potential lapses or cancellations. This enabled the company to act early with tailored communications and offers. The approach also focused on creating a seamless, personalized customer experience. The implementation of predictive analytics led to better engagement with high-risk policies, preventing lapses and enhancing customer satisfaction. As a result, the company saw improved retention rates, reduced cancellations, and increased profitability.
How We Did It
Fuld & Company implemented a cutting-edge predictive analytics model powered by machine learning to forecast policy risks and reduce churn. Here’s how we made it happen:
- Data Collection: Consolidated data from vehicle finance groups, sales, and collection records to create a robust dataset.
- Feature Engineering: Addressed missing data, normalized variables, and encoded categories to ensure accuracy in modeling.
- Model Development: Built an ensemble model using XGBoost, achieving exceptional accuracy in predicting policy lapses.
- Implementation: Seamlessly integrated the model with the insurer’s CRM for real-time monitoring, personalized risk assessment, and actionable insights.
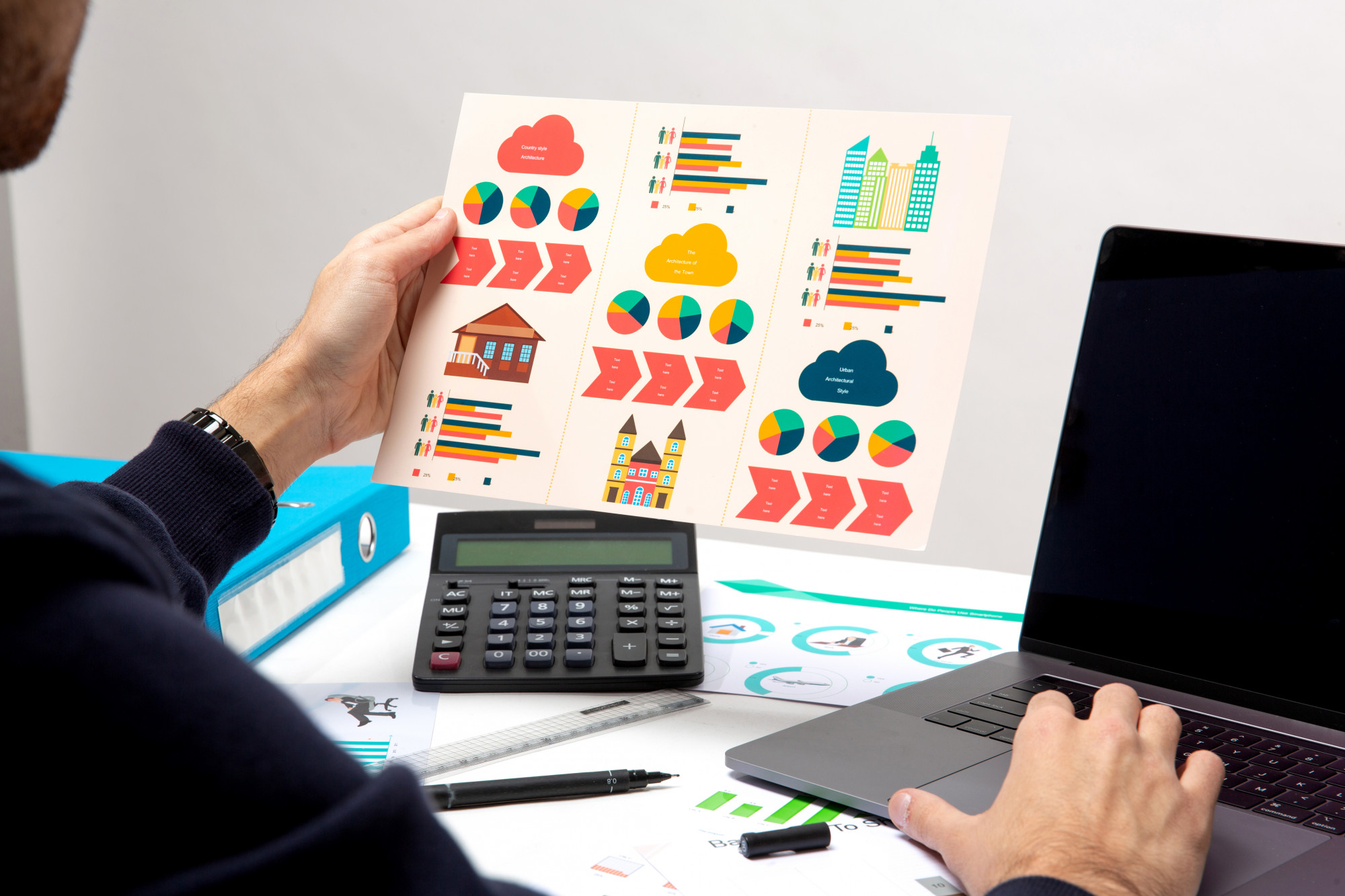