Machine Learning-Driven Demand Planning and Forecasting Increases Retail Profitability for US Retail Giant
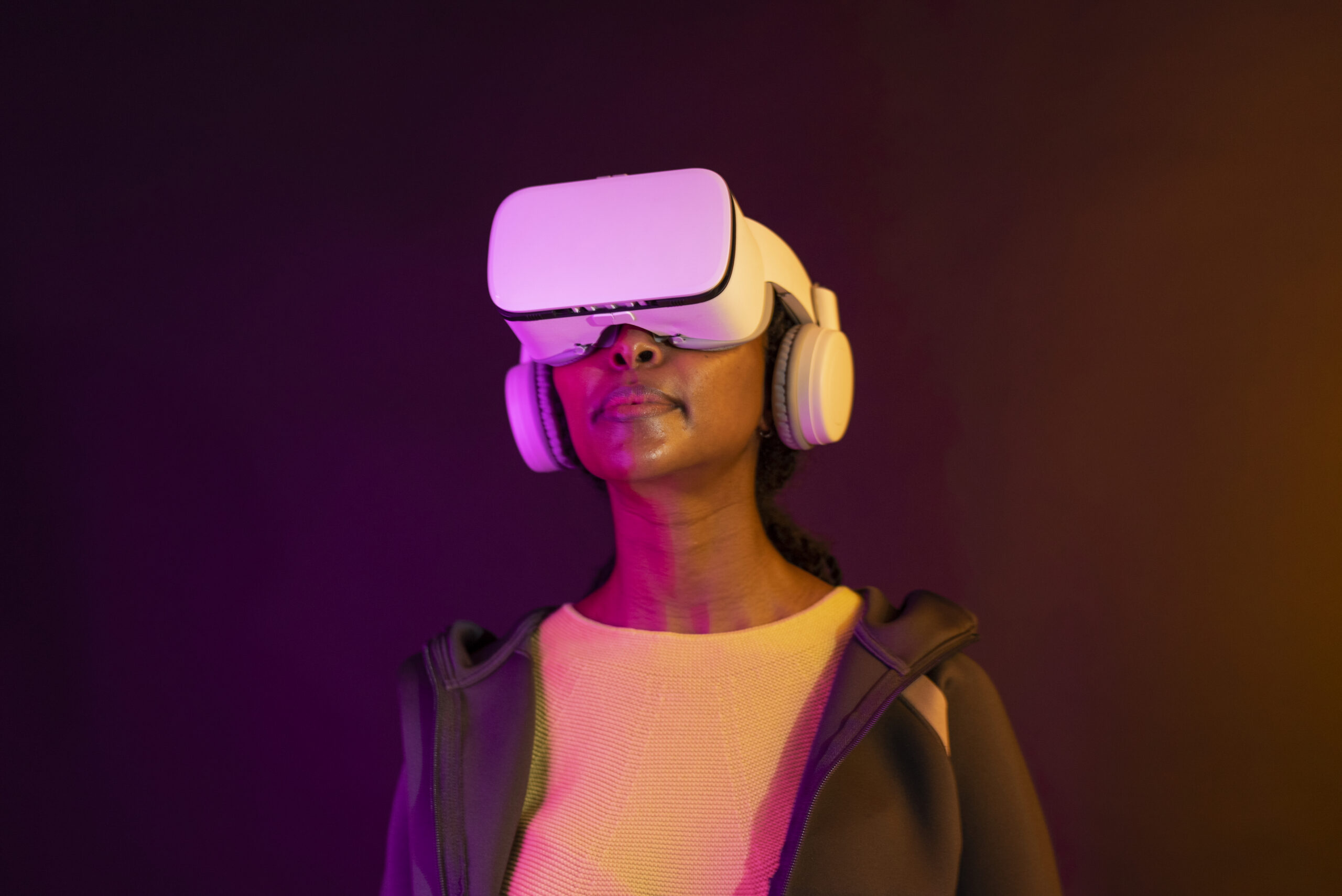
A major retailer aimed to enhance its demand planning processes and optimize pricing strategies to boost sales and profitability. Fuld & Company used advanced analytics to better understand how price changes impact demand, forecast weekly sales, and refine pricing decisions across stores. The goal was to ensure the right products were available at the right price.
Fuld implemented machine learning-driven demand planning and forecasting to deliver the following results:
- 1200+ Models Trained: Over 1200 machine learning models were trained to fine-tune pricing, demand forecasting, and sales predictions, ensuring accurate, data-driven insights.
- 100+ Stores Analyzed: Performance across more than 100 stores was analyzed to account for regional variations, ensuring that pricing and demand forecasts were applied at a granular level.
- Increased Profitability: By optimizing pricing and anticipating demand fluctuations, the retailer experienced improved profit margins, sales growth, and inventory efficiency.
How We Did It
Fuld & Company deployed a sophisticated machine learning model that utilized several key components to improve the retailer’s demand forecasting and pricing strategies:
- Price-Demand Response Curve Modeling
We applied machine learning algorithms to model the price-demand response curve, enabling the retailer to understand how changes in price affected consumer demand for different products. This model helped identify optimal pricing strategies to maximize sales while maintaining profitability.
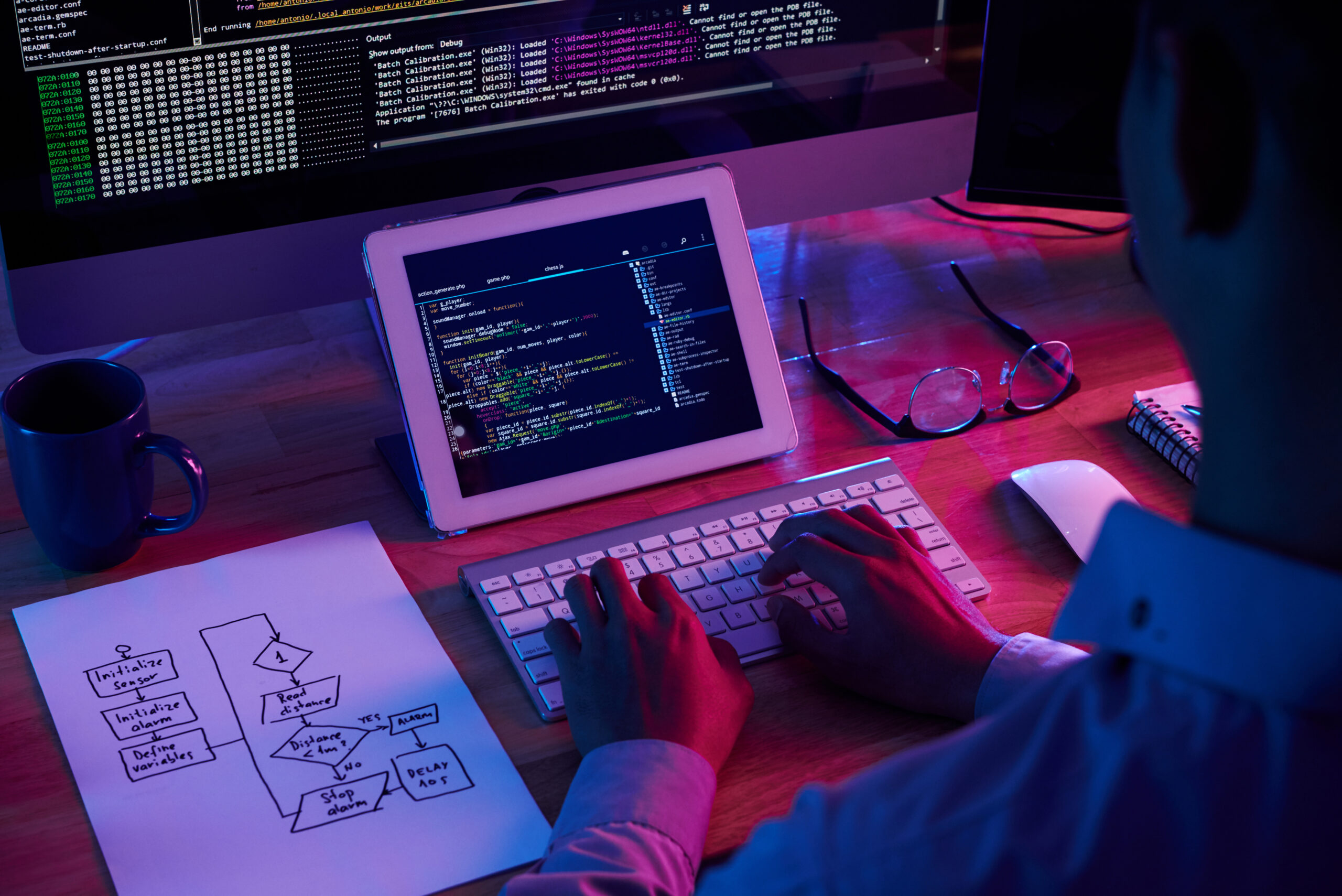
- Store-Level Pricing Optimization
Our machine learning model accounted for regional differences in demand, competition, and consumer behavior across various store locations. By analyzing historical data and store-specific factors, we determined optimal pricing for each item and store, ensuring competitive and profitable pricing strategies at a localized level.
- Weekly Sales and Profit Forecasting
We developed predictive models to forecast weekly sales and profits based on historical data, seasonal trends, and other influencing factors. These forecasts allowed the retailer to gain early performance signals, enabling them to make informed decisions about inventory and promotions ahead of time.
- Early Performance Signals for Smarter Decision-Making
By providing early performance signals, the retailer could adjust pricing, inventory, and promotional strategies before issues like stock-outs or slow-moving inventory became significant problems. This helped optimize both sales and profit margins across the company’s broad network of stores.